Dynamic Autonomy and Intelligent Robotics Lab
170B Towne Building, 220 S. 33rd Street, Philadelphia PA, 19104
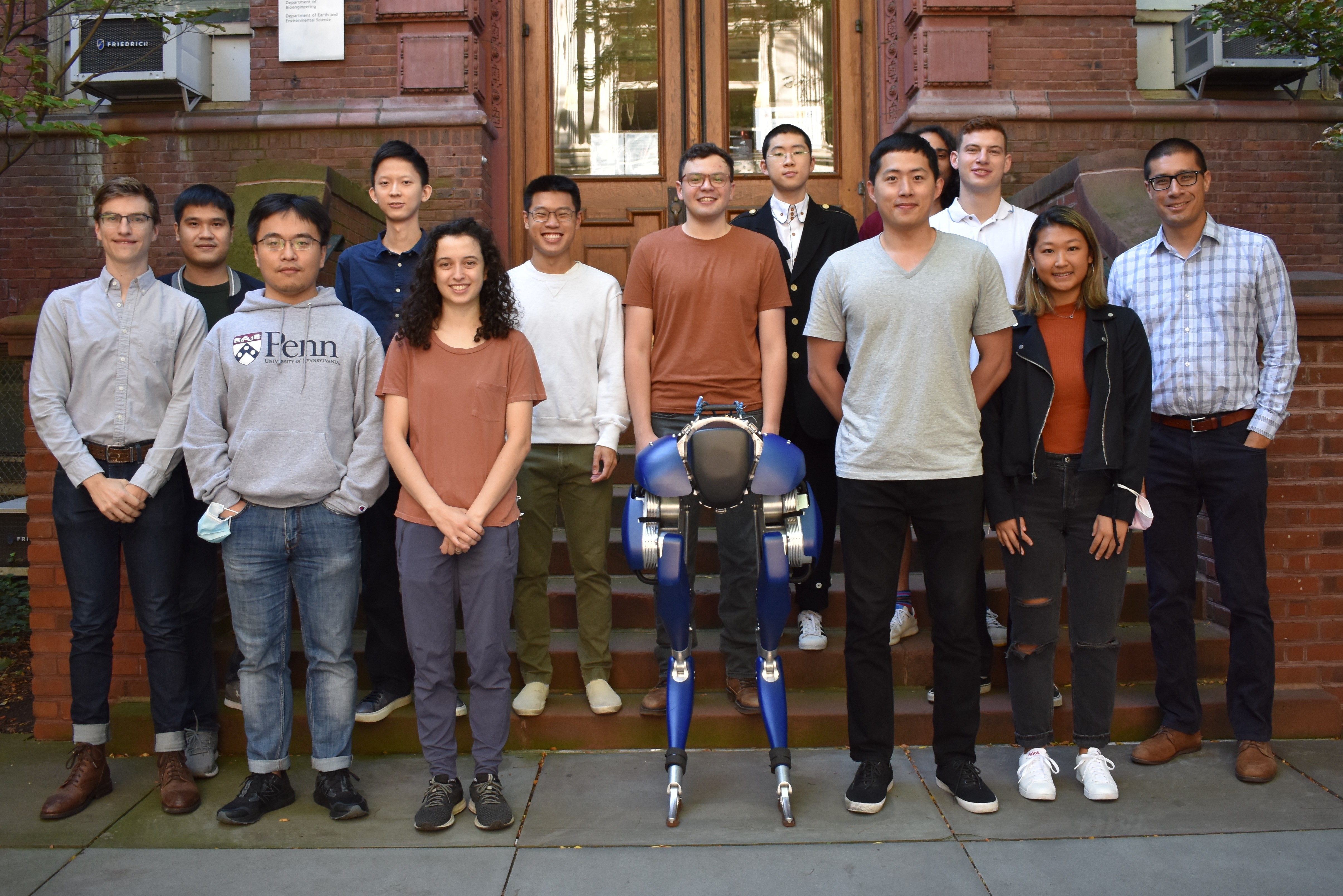
Welcome to the Dynamic Autonomy and Intelligent Robotics (DAIR) Lab!
Our research centers on control, learning, planning, and analysis of robots as they interact with the world. Whether a robot is assisting within the home, or operating in a manufacturing plant, the fundamental promise of robotics requires touching and affecting a complex environment in a safe and controlled fashion. We are focused on developing computationally tractable and data efficient algorithms which enable robots to operate both dynamically and safely as they quickly maneuver through and interact with their environments.
Right now, we are particularly interested in understanding the interplay between the non-smooth dynamics of contact, machine learning, and numerical optimization, and then testing these techniques on both legged robots and robotic manipulation.
For more information on our work, please see Michael’s research statement.
We are proud to be a group within the Penn Engineering GRASP Lab.
Recent Updates
May 21, 2025 |
The DAIR Lab will be participating in three workshops on Friday at ICRA 2025. Come say hello!
|
---|---|
May 16, 2025 | Congratulations to Brian Acosta, who successfully defended his thesis, titled “Real-time Perception and Mixed-Integer Footstep Control for Underactuated Bipedal Walking on Rough Terrain.” Brian’s thesis was a beautiful synthesis of full-stack bipedal robots: he developed new strategies for robust extraction of walkable terrain from depth data and algorithms for combined foostep planning and dynamic control. Check out the recording below, with the document itself to come soon! |
Apr 18, 2025 | We are honored to receive the 2024 Best Paper Award from the IEEE RAS TC on Model-based Optimization for Robotics, for our T-RO paper “Consensus Complementarity Control for Multi-Contact MPC.” Congratulations to DAIR alumni Alp Aydinoglu, Wei-Cheng Huang, and Adam Wei! |
Mar 30, 2025 | Congratulations to Bibit Bianchini for being awarded the 2025 John Goff Prize. The Goff Prize is awarded annually to one or two MEAM graduate students at Penn on the basis of criteria of scholarship, resourcefulness, and leadership. |
Dec 31, 2024 | Congratulations to Bibit Bianchini for being selected to the 2024 Rising Stars in Mechanical Engineering Workshop, held in October at CMU! |
Sep 5, 2024 | Congratulations to William Yang, who successfully defended his thesis, titled “Controlling Contact Transitions for Dynamic Robots.” Will demonstrated remarkable new robot capabilities for controlling both high-speed impact events for bipedal running and jumping, and for dynamic, dexterous manipulation exploiting sliding friction and stick-slip transitions. The thesis document itself will be uploaded soon. |
Aug 1, 2024 | For the upcoming 2024-2025 application cycle, we will be looking to recruit multiple incoming Ph.D. students across all relevant departments (MEAM, ESE, or CIS). We are dedicated to assembling a dynamic and diverse team of researchers, and actively seek individuals with diverse cultural, ethnic, socioeconomic, and academic backgrounds. Get more information and apply here. |
Jul 19, 2024 | Congratulations to Will Yang! Will’s paper, “Dynamic On-Palm Manipulation via Controlled Sliding,” received the Outstanding Student Paper Award at Robotics: Science and Systems (RSS)! |
May 22, 2024 | How far can you get by simply specifying that an object move? With contact-implicit MPC, quite far! Will Yang’s paper “Dynamic On-Palm Manipulation via Controlled Sliding,” accepted to RSS 2024, pushes the limits of real-time dexterity. Check out the project website and arXiv preprint |
May 2, 2024 |
We have another recently published paper in IEEE Transactions on Robotics!
Simple models, like inverted pendulums and single rigid-body models, are ubiquitous in legged locomotion. They achieve good performance with only a low-dimensional representation, but can an algorithm do better? We explore the use of numerical optimization to synthesize new simple models, achieving higher performance across a wider range of bipedal walking tasks.
This work led by recent grad Yu-Ming Chen, and supported by the Toyota Research Institute. Check out the project website, paper, and freely available arXiv version |
Lab Wiki (private)